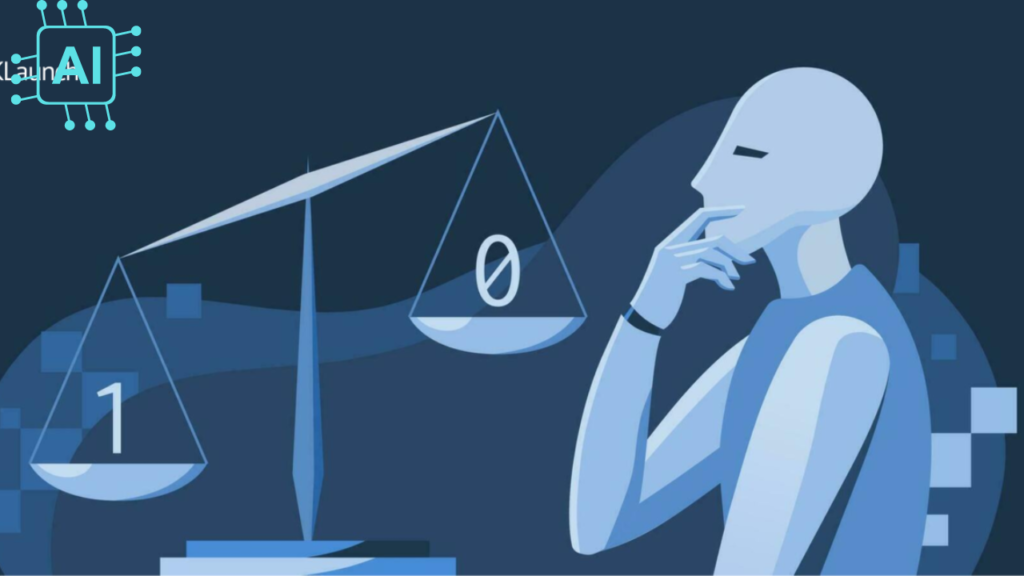
Artificial Intelligence (AI) has become an integral part of our lives, influencing decisions across a wide range of sectors—from healthcare to finance, and even criminal justice. As AI systems continue to evolve and integrate into these critical areas, the importance of ensuring fairness and accountability in AI decision-making cannot be overstated. This article delves into the challenges and strategies associated with making AI systems fair, transparent, and accountable.
The Growing Influence of AI in Decision-Making
AI is increasingly being used to make decisions that affect people’s lives. From credit scoring algorithms that determine loan eligibility to predictive policing systems that assess crime risks, AI’s reach is expanding rapidly. While these systems offer efficiency and the potential to eliminate human biases, they also pose significant risks if not carefully managed. Bias in AI algorithms can lead to unfair treatment of individuals or groups, and the lack of transparency can make it difficult to understand or challenge decisions made by AI.
Understanding Fairness in AI
What is Fairness in AI?
Fairness in AI refers to the principle that AI systems should make decisions that are free from bias and do not unfairly discriminate against any individual or group. This is particularly challenging because AI systems learn from historical data that may reflect existing biases. If these biases are not addressed, they can be perpetuated and even amplified by AI.
Types of Bias in AI
There are several types of bias that can affect AI decision-making, including:
- Data Bias: This occurs when the data used to train an AI model is not representative of the population it is meant to serve. For example, if an AI system is trained on data that underrepresents certain demographic groups, it may make biased decisions that disadvantage those groups.
- Algorithmic Bias: This happens when the algorithms themselves, or the way they are designed, introduce bias into the decision-making process. This can occur even if the data is unbiased.
- Bias in Interpretation: Even if an AI system is trained on fair data and uses unbiased algorithms, the way its results are interpreted by humans can introduce bias.
Strategies for Ensuring Fairness in AI
1. Diverse and Representative Data
One of the most effective ways to ensure fairness in AI is to use diverse and representative data when training AI models. This means including data from a wide range of sources and ensuring that all relevant demographic groups are adequately represented. It also involves continually updating the data to reflect changes in society.
2. Algorithmic Transparency
Transparency in how AI algorithms are designed and how they operate is crucial for fairness. Algorithmic transparency allows stakeholders to understand how decisions are made and to identify any potential biases. This can be achieved through the use of explainable AI (XAI), which provides insights into how AI models make decisions.
3. Regular Audits and Monitoring
Regular audits and monitoring of AI systems are essential for identifying and correcting biases. AI audits should be conducted by independent third parties to ensure objectivity. These audits should evaluate both the data used to train the AI and the algorithms themselves.
4. Inclusive Design and Development
AI systems should be designed and developed with input from a diverse group of stakeholders, including those from underrepresented groups. Inclusive design ensures that the perspectives and needs of all users are considered, reducing the risk of bias.
5. Ethical Guidelines and Regulations
Governments and organizations should establish ethical guidelines and regulations to govern the development and use of AI. These guidelines should include principles for fairness, transparency, and accountability, and should be enforced through legislation and oversight bodies.
Accountability in AI Decision-Making
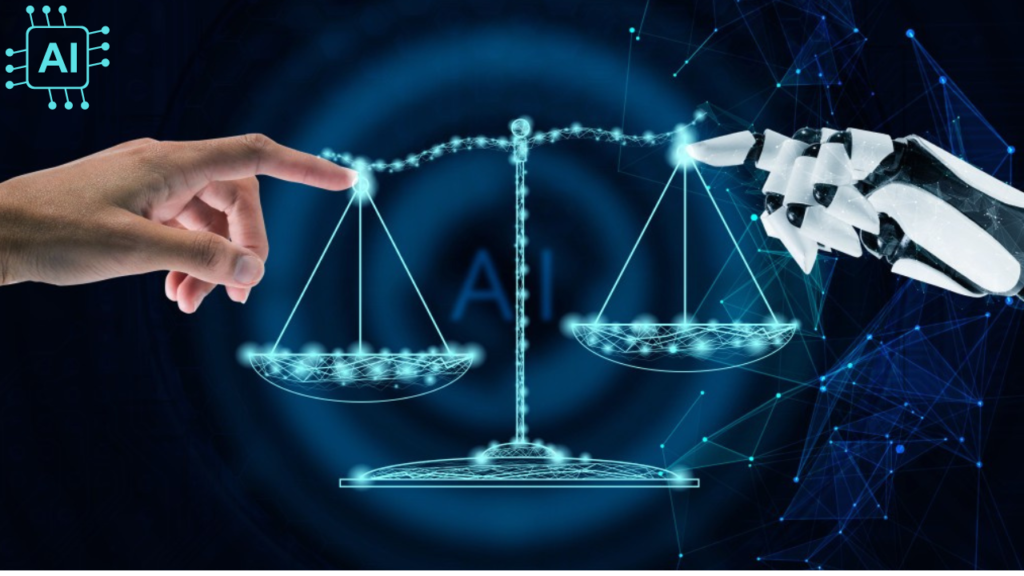
The Importance of Accountability
Accountability in AI decision-making is critical to maintaining public trust. When AI systems make decisions that affect people’s lives, there must be mechanisms in place to hold those systems, and the organizations that deploy them, accountable for their actions.
Challenges in AI Accountability
Ensuring accountability in AI is challenging due to the complexity and opacity of many AI systems. Black-box algorithms—those whose internal workings are not visible or understandable to humans—pose particular challenges. When decisions are made by these systems, it can be difficult to trace back the reasoning behind them, making accountability difficult to enforce.
Strategies for Enhancing Accountability
1. Clear Documentation and Record-Keeping: Organizations should maintain detailed records of how AI systems are designed, trained, and deployed. This documentation should include information on the data used, the algorithms selected, and the decision-making process.
2. Human Oversight: While AI can make decisions autonomously, there should always be a level of human oversight to review and, if necessary, override AI decisions. This helps to ensure that AI systems do not operate in a vacuum and that there is a human element involved in the decision-making process.
3. Legal and Regulatory Frameworks: Governments should establish legal frameworks that hold organizations accountable for the decisions made by their AI systems. This includes establishing penalties for harm caused by biased or unfair AI decisions and creating mechanisms for individuals to challenge AI decisions that they believe are unfair.
4. Third-Party Audits: As with fairness, third-party audits are essential for accountability. Independent audits can provide an objective assessment of an AI system’s fairness and accountability, ensuring that organizations adhere to best practices.
The Future of Fair and Accountable AI
As AI continues to advance, ensuring fairness and accountability in AI decision-making will become even more critical. The stakes are high, and the potential for harm is significant if these issues are not addressed. However, by implementing the strategies outlined in this article, organizations can develop AI systems that are both fair and accountable, ultimately benefiting society as a whole.