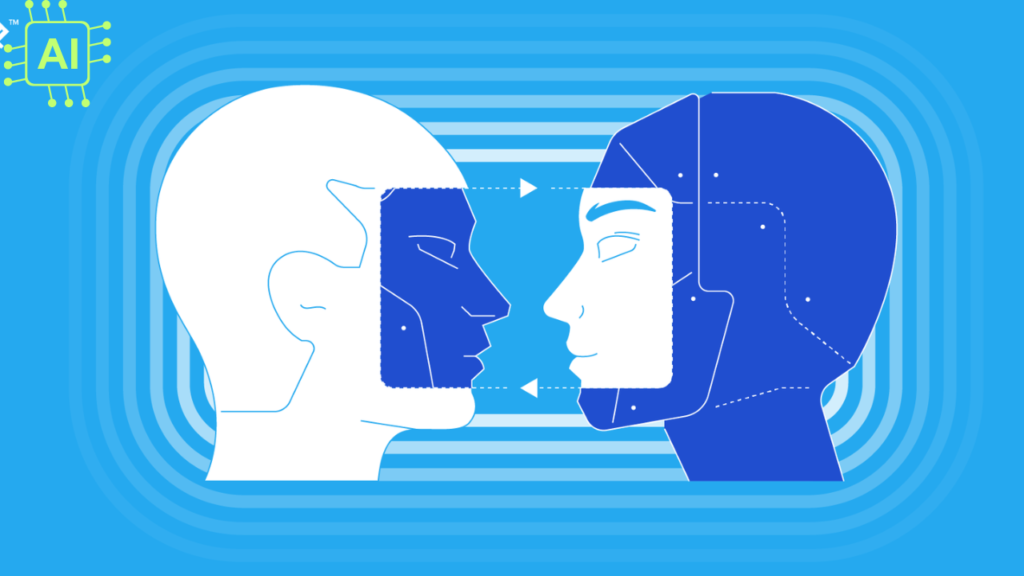
In the rapidly evolving landscape of artificial intelligence, we find ourselves grappling with a critical issue that threatens the fairness and effectiveness of AI systems: AI bias. As we delve into this complex topic, we’ll explore the intricacies of AI bias, its origins, and most importantly, how we can work towards mitigating its effects to create more equitable algorithms.
The Nature of AI Bias
AI bias refers to the systematic and repeatable errors in a computer system that create unfair outcomes, such as privileging one arbitrary group of users over others. These biases can manifest in various ways, from facial recognition systems that struggle to identify people of color to hiring algorithms that favor male candidates over equally qualified female applicants.
The root of AI bias often lies in the data used to train these systems. If the training data is not representative of the diverse population it’s meant to serve, the resulting AI model will inevitably reflect and amplify these imbalances. This is why we must be vigilant in our approach to data collection and curation.
Sources of AI Bias
Understanding the origins of AI bias is crucial for developing effective mitigation strategies. Some common sources include:
- Historical bias: When the data used to train AI systems reflects past prejudices and unfair practices.
- Representation bias: Occurs when certain groups are underrepresented in the training data.
- Measurement bias: Arises from the way data is collected or measured, which may favor certain groups over others.
- Aggregation bias: When models are applied across diverse groups without accounting for important differences between them.
- Evaluation bias: Stems from the benchmarks and metrics used to evaluate AI systems, which may not capture real-world performance across all groups.
The Impact of AI Bias
The consequences of AI bias can be far-reaching and profound. In healthcare, biased algorithms might lead to misdiagnoses or unequal access to treatment. In criminal justice, they could perpetuate systemic racism through unfair sentencing recommendations. In finance, biased AI could deny loans to qualified individuals from marginalized communities.
These outcomes not only harm individuals but also erode trust in AI systems and hinder their potential to drive positive change in society. As we continue to integrate AI into critical decision-making processes, addressing bias becomes not just a technical challenge but an ethical imperative.
Strategies for Mitigating AI Bias
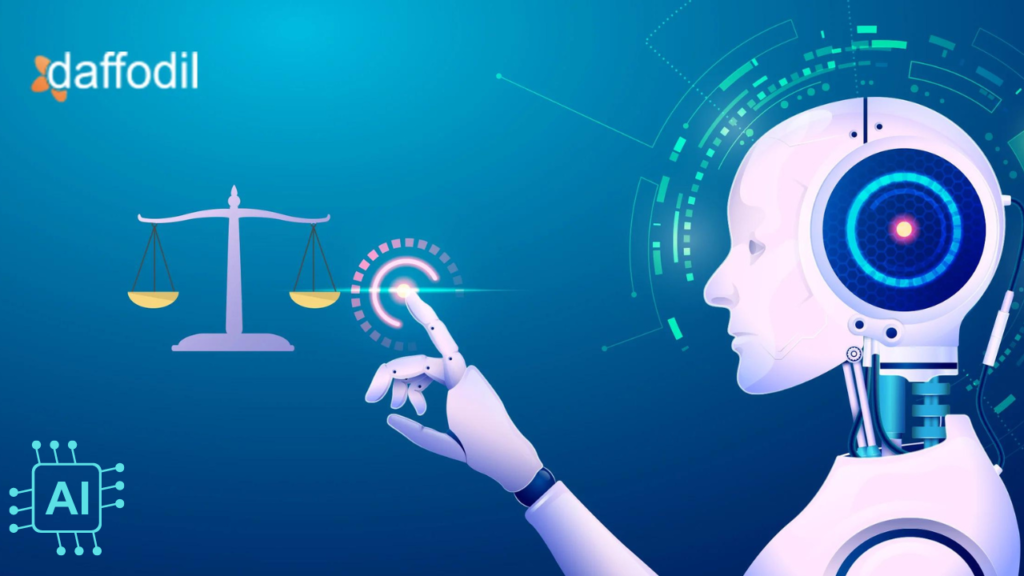
Mitigating AI bias requires a multi-faceted approach that combines technical solutions with ethical considerations and diverse perspectives. Here are some key strategies we can employ:
1. Diverse and Representative Data
Ensuring that training data is diverse and representative of the population the AI system will serve is crucial. This means actively seeking out data from underrepresented groups and carefully balancing datasets to avoid overrepresentation of majority groups.
2. Rigorous Testing and Auditing
Implementing comprehensive testing protocols that specifically look for biases across different demographic groups is essential. This includes regular audits of AI systems in production to identify and address any emergent biases.
3. Transparent AI Systems
Developing AI models that are interpretable and explainable can help in identifying the root causes of biases. This transparency also builds trust with users and allows for more effective oversight.
4. Diverse Development Teams
Fostering diversity within AI development teams brings a wider range of perspectives and experiences to the table, helping to identify potential biases that might otherwise go unnoticed.
5. Ethical Guidelines and Governance
Establishing clear ethical guidelines for AI development and deployment, along with robust governance structures, can help ensure that bias mitigation remains a priority throughout the AI lifecycle.
6. Continual Monitoring and Improvement
Recognizing that bias mitigation is an ongoing process, not a one-time fix, is crucial. Continual monitoring of AI systems in real-world applications allows for iterative improvements and adjustments.
The Role of Regulation in Addressing AI Bias
As the impact of AI on society grows, the role of regulation in addressing AI bias becomes increasingly important. Governments and regulatory bodies around the world are beginning to develop frameworks to ensure the responsible development and deployment of AI systems.
These regulations aim to:
- Mandate transparency in AI decision-making processes
- Require regular audits of AI systems for potential biases
- Establish accountability measures for companies deploying AI technologies
- Protect individuals’ rights in the face of automated decision-making
While the specifics of these regulations are still evolving, they signal a growing recognition of the need for oversight in the AI industry to protect against unfair and discriminatory practices.
The Future of Fair AI
As we look to the future, the pursuit of fair and unbiased AI systems remains a critical challenge and opportunity. Advances in techniques such as federated learning, which allows for model training on distributed datasets without centralizing sensitive information, offer promising avenues for developing more inclusive AI systems while protecting privacy.
Moreover, the growing field of AI ethics is fostering important discussions about the values we want to embed in our AI systems and how we can align technological progress with societal good. By continuing to prioritize fairness, transparency, and inclusivity in AI development, we can work towards a future where AI technologies enhance human potential and promote equality rather than exacerbate existing disparities.
Conclusion
Understanding and mitigating AI bias is not just a technical challenge but a societal imperative. As AI systems become increasingly integrated into our daily lives and decision-making processes, ensuring their fairness and equity is crucial for building a just and inclusive future.
By implementing robust strategies for bias mitigation, fostering diverse and inclusive development practices, and engaging in ongoing dialogue about the ethical implications of AI, we can work towards creating AI systems that truly serve all members of society. The journey towards fair AI is complex and ongoing, but it is a journey we must undertake to harness the full potential of artificial intelligence for the betterment of humanity.